Differentiation is no longer about who can collect the most data. It’s about who can quickly make sense of the data they collect. There once was a time when hardware sampling rates, limited by the speed at which analog-to-digital conversion took place, physically restricted how much data was acquired. But today, hardware is no longer the limiting factor in acquisition applications. The management of acquired data is the challenge of the future.
收集更多的数据已经不能让您脱引而出,更重要的是谁能够迅速分清所收集到的数据。 在过去,硬件采样率由于受模数转换发生速度的限制,在物理上局限了采集数据的数量。 而如今,硬件已不再是采集应用的限制因素。 如何管理采集到的数据才是未来的挑战。
Advances in computing technology, including increasing microprocessor speed and hard-drive storage capacity, combined with decreasing costs for hardware and software have provoked an explosion of data coming in at a blistering pace. In measurement applications in particular, engineers and scientists can collect vast amounts of data every second of every day. For every second that the Large Hadron Collider at CERN runs an experiment, the instrument generates 40 terabytes of data. For every 30 minutes that a Boeing jet engine runs, the system creates 10 terabytes of operations information (Gantz, 2011). That’s “big data.”
计算技术的不断进步,包括了微处理器速度和硬盘驱动器存储容量的提高,加之软硬件成本的降低,引发了惊人速度的数据爆炸。 特别是在测量应用中,工程师和科学家们每分每秒都能收集大量的数据。 欧洲核子研究中心的大型强子对撞机的运行实验每秒钟能产生40 TB的数据。 而波音喷气发动机运行时,每隔30分钟系就统会创建10 TB的操作信息(Gantz,2011)。 这就是“大规模数据”。
The big data phenomenon adds new challenges to data analysis, search, integration, reporting, and system maintenance that must be met to keep pace with the exponential growth of data. And the sources of data are many. However, among the most interesting to the engineer and scientist is data derived from the physical world. This is analog data that is captured and digitized. Thus, it can be called “Big Analog Data.” It is collected from measurements of vibration, RF signals, temperature, pressure, sound, image, light, magnetism, voltage, and so on. Challenges unique to Big Analog DataTM have provoked three technology trends in the widespread field of data acquisition.
大规模数据现象为数据分析、搜索、集成、报告和系统维护带来了新的挑战,只有满足这些挑战才能跟上数据飞速增长的步伐。 数据的来源是多方面的,而工程师和科学家认为*为有趣的是来自真实世界的数据, 即捕获和数字化的测量数据。 因此,它也被称作“大规模测量数据”,可以通过测量振动、射频信号、温度、压力、声音、图象、光、磁、电压等现象获得这些数据。 大规模测量数据TM在广泛的数据采集领域激起了三大技术趋势。
Contextual Data Mining
上下文数据挖掘
The physical characteristics of some real-world phenomena prevent information from being gleaned unless acquisition rates are high enough, which makes small data sets an impossibility. Even when the characteristics of the measured phenomena allow more information gathering, small data sets often limit the accuracy of conclusions and predictions in the first place.
真实现象的物理特性能够防止在采集速率不够高的时候采集数据,让小规模数据集变得不再可行。 即使测量现象的特性允许更多的信息采集,小规模数据集往往一开始就限制了结论和预测的准确性。
Consider a gold mine where only 20% of the gold is visible. The remaining 80% is in the dirt where you can’t see it. Mining is required to realize the full value of the contents of the mine. This leads to the term “digital dirt,” meaning digitized data can have concealed value. Hence, data analytics and data mining are required to achieve new insights that have never before been seen.
以挖掘一个金矿为例,其中只有20%的黄金是可见的。 其余的80%是存在于您看不见的泥土中。 矿业的目的就是充分挖掘矿井的全部价值。 这就引出了术语“数字尘土”,意思为数字化数据带有隐藏价值。 因此,需要通过数据分析和数据挖掘来发掘****的见解。
Data mining is the practice of using the contextual information saved along with data to search through and pare down large data sets into more manageable, applicable volumes. By storing raw data alongside its original context, or “metadata,” it becomes easier to accumulate, locate, and later manipulate and understand. For example, examine a series of seemingly random integers: 5126838937. At first glance, it is impossible to make sense of this raw information. However, when given context like (512) 683-8937, the data is much easier to recognize and interpret as a phone number.
数据挖掘的过程就是使用与数据一同保存的上下文信息,搜索并削减大规模数据集,使其变得更容易管理及利用。 将原始数据与背景,或“元数据”共同保存下来,数据采集、定位、过后的处理和理解就会变得更为方便。 例如,查看一系列看似随机的整数: 5126838937。乍看之下,该原始信息的含义不得而知。 然而,当它变为(512)683-8937时,我们就能知道清楚地识别出它是一个电话号码。
Descriptive information about measurement data context provides the same benefits and can detail anything from sensor type, manufacturer, or calibration date for a given measurement channel to revision, designer, or model number for an overall component under test. In fact, the more context that is stored with raw data, the more effectively that data can be traced throughout the design life cycle, searched for or located, and correlated with other measurements in the future by dedicated data post-processing software.
测量数据上下文的描述性信息提供了类似的益处,它能够详细描述指定测量通道的传感器类型、制造商与校准日期,或是整体待测组件的修订记录、设计师或型号。 事实上,原始数据存储的上下文越多,在整个设计生命周期中数据追踪、搜索或定位,以及通过专用数据后处理软件在今后与其他测量关联才会更为有效。
Intelligent DAQ Nodes
智能DAQ节点
Data acquisition applications are incredibly diverse. But across a wide variety of industries and applications, data is rarely acquired simply for the sake of acquiring it. Engineers and scientists invest critical resources into building advanced acquisition systems, but the raw data produced by those systems is not the end game. Instead, raw data is collected so it can be used as an input to analysis or processing algorithms that lead to the actual results system designers seek.
数据采集应用的形式多种多样。 但由于涉及多种行业和应用,只有在需要时才会采集数据。 工程师和科学家们将重要资源投资在构建**采集系统上,但这些系统生成的原始数据也不会因此就无用了。 相反,采集原始数据,将它输入分析或处理算法,构建设计者所需的实际结果系统。
For example, automotive crash tests can collect gigabytes of data in a few tenths of a second that represent speeds, temperatures, forces of impact, and acceleration. But one of the key pieces of pertinent knowledge that can be computed from this raw data is the Head Injury Criterion (HIC), a single scalar, calculated value representing the likelihood of a crash dummy to experience a head injury in the crash.
例如,汽车碰撞测试在毫秒之间就能收集千兆字节有关速度、温度、冲击力和加速度的数据。 可以从这些原始数据计算得出的一个关键性相关结论为颅脑损伤标准(HIC),它是单标量的计算值,能够表示碰撞假人在碰撞中头部受伤的可能性。
Additionally, some applications—particularly in the environmental, structural, or machine condition monitoring spaces—avail themselves to periodic, slow acquisition rates that can be drastically increased in bursts when a noteworthy condition is detected. This technique keeps acquisition speeds low and minimizes logged data while allowing sampling rates that are adequate enough for high-speed waveforms when necessary in these applications. To incorporate tactics such as processing raw data into results or adjusting measurement details when certain criteria are met, you must integrate intelligence into the data-acquisition system.
此外,一些应用程序—尤其是有关环境、结构、机器状态监测空间—能够保持周期性的慢采集速率,而当检测到明显的条件时又能大幅提高。 该技术的采集速度低,且*大限度地减少了记录的数据,同时采样率要足够满足应用中高速波形的需求。 想要在满足特定标准时,采用某项技术,如将原始数据转化为结果,或调整测量细节,您必须使您的数据采集系统智能化。
Though it’s common to stream test data to a host PC (the “intelligence”) over standard buses like USB and Ethernet, high-channel-count measurements with fast sampling rates can easily overload the communication bus. An alternative approach is to store data locally and transfer files for post-processing after a test is run, which increases the time it takes to realize valuable results. To overcome these challenges, the latest measurement systems integrate leading technology from ARM, Intel, and Xilinx to offer increased performance and processing capabilities as well as off-the-shelf storage components to provide high-throughput streaming to disk.
虽然将测试数据通过标准总线,如USB和以太网,传输到PC主机上(“智能”)非常常见,但是高通道数测量的采样速率非常快,很可能超过通信总线的负荷。另一种方法是在本地存储数据,在测试运行后传输文件进行后期处理,所花费的时间会增加,但能获得有价值的结果。 为了应对这些挑战,*新的测量系统集成了来自ARM、Intel和Xilinx的**技术,可提供更高的性能和处理能力,以及现成的存储组件,以提供高通量数据传输。
With onboard processors, the intelligence of measurement systems has become more decentralized by having processing elements closer to the sensor and the measurement itself. Modern data acquisition hardware includes high-performance multicore processors that can run acquisition software and processing-intensive analysis algorithms in line with the measurements. These intelligent measurement systems can analyze and deliver results more quickly without waiting for large amounts of data to transfer, or without having to log it in the first place, which optimizes the system to use disk space more efficiently.
借助板载处理器,处理元件更接近传感器和测量,测量系统的数据就变得更为分散。 现代数据采集硬件包含了高性能的多核处理器,可以根据测量运行采集软件和处理密集型分析算法。 这些智能测量系统能够更快地分析并得出结论,无需花费时间再等待大量的传输数据,也无需立即记录数据,优化了系统,从而更有效地利用磁盘空间。
The Rise of Cloud Storage and Computing
云存储和计算的崛起
The unification of DAQ hardware and onboard intelligence has enabled systems to be increasingly embedded or remote. In many industries, it has paved the way for entirely new applications. As a result, the Internet of Things is finally unfolding before our very eyes as the physical world is embedded with intelligence and humans now can collect data sets about virtually any environment around them. The ability to process and analyze these new data sets about the physical world will have profound effects across a massive array of industries. From health care to energy generation, from transportation to fitness equipment, and from building automation to insurance, the possibilities are virtually endless.
DAQ硬件和板载智能化的统一可创建出进一步嵌入式或远程系统。 在许多行业,它解决了众多全新应用的难题。 真实世界正变得智能化,人类现在也能够收集几乎所有周围环境的数据集,物联网因而出现在了我们眼前。 它能够处理并分析真实世界新数据集,将对众多行业领域产生深远的影响,医疗、能源、交通、健身器材、楼宇自动化、保险,它可谓无处不在。
In most of these industries, content (or the data collected) is not the problem. There are plenty of smart people collecting lots of useful data out there. To date this has mainly been an IT problem. The Internet of Things is generating massive amounts of data from remote, field-based equipment spread literally across the world and sometimes in the most remote and inhospitable environments.
在大部分的行业中,内容自身 (所采集的数据)并非问题的根本。 聪明的人们收集了大量有用的数据。 至目前为止,主要的问题还是出在IT上面。 物联网通过分布在世界各地的远程现场设备产生大量数据,有些数据还可能来自*偏远、荒凉的地区。
These distributed acquisition and analysis nodes (DAANs) embedded in other end products are effectively computer systems with software drivers and images that often connect to several computer networks in parallel. They form some of the most complex distributed systems and generate some of the largest data sets the world has ever seen. These systems need remote network-based systems management tools to automate the configurations, maintenance, and upgrades of the DAANs and a way to efficiently and cost-effectively process all of that data.
这些分布式采集和分析节点(DAAN)嵌入在其他终端产品中,软件驱动程序和图像并行连接至多个计算机网络,形成了计算机系统。 他们形成了*为复杂的分布式系统,生成了史上*大的数据集。 这些系统需要基于远程网络的系统管理工具来自动配置、维护及升级DAANs,并需要一种高效、低成本的方式来处理所有数据。
Complicating matters is that if you reduce the traditional IT topology for most of the organizations collecting such data to a simple form, you find they are actually running two parallel networks of distributed systems: “the embedded network” that is connected to all of the field devices (DAANs) collecting the data and “the traditional IT network” where the most useful data analysis is implemented and distributed to users.
问题的复杂在于,如果您减少数据采集传统的IT拓扑结构,简化形式,就会发现他们实际上运行在两个并行的分布式系统网络上: “嵌入式网络”连接到所有采集数据的现场设备(DAAN), “传统IT网络”,进行*有用的数据分析,并传送给用户。
More often than not, there is a massive fracture between these two parallel networks within organizations, and they are incapable of interoperating. This means that the data sets cannot get to the point(s) where they are most useful. Think of the power an oil and gas company could achieve by collecting real-time data on the amount of oil coming out of the ground and running through a pipeline in Alaska and then being able to get that data to the accounting department, the purchasing department, the logistics department, or the financial department—all located in Houston—within minutes or hours instead of days or months.
而往往,这两个并行的组织网络内存在巨大的差异,它们不能互相操作。 这意味着,数据集的效果发挥至**。 石油和天然气公司收集地下生成以及通过阿拉斯加管道石油量的实时数据,将这些数据交给会计部门、采购部门、物流部门或财政部门——他们都位于休斯顿——这一切在数分钟或数小时内就能完成,省去了成天甚至成月的时间。
The existence of parallel networks within organizations and the major investment made in them have been major inhibitors for the Internet of Things. However, today cloud storage, cloud computational power, and cloud-based “big data” tools have met these challenges. It is simple to use cloud storage and cloud computing resources to create a single aggregation point for data coming in from a large number of embedded devices (such as the DAANs) and provide access to that data from any group within the organization. This solves the problem of the two parallel embedded and IT networks that don’t interoperate.
组织内的并行网络和其中大量的投资大大抑制了物联网的发展。 而如今,通过云存储、云计算,以及基于云的“海量数据”工具,这些难题都能够得到解决。 使用云存储和云计算资源创建单个汇聚点非常容易,它能够汇集来自嵌入式设备(例如DAAN)的大量数据,且组织内的任何一组都能访问这些数据。 这就解决了两个并行嵌入式与IT网络不能相互操作的问题。
Placing near infinite storage and computing resources from the cloud that are used and billed on-demand at the fingertips of users provides solutions to the challenges of distributed system management and crunching huge data sets of acquired measurement data. Big data tool suites offered by cloud providers make it easy to ingest and make sense of these huge measurement data sets.
用户可以使用云中无限的存储和计算资源,并根据使用量计费,这为他们提供了管理分布式系统以及处理大型测量数据集的解决方案。 云服务供应商所提供的海量数据工具套件,可以帮助用户轻松的获取并处理这些庞大的测量数据集。
To summarize, cloud technologies offer three broad benefits for distributed system management and data access: aggregation of data, access to data, and offloading of computationally heavy tasks.
总的说来,云计算技术为分布式系统管理和数据访问带来了三大好处: 数据的汇总、访问数据,以及卸载计算繁重的任务。
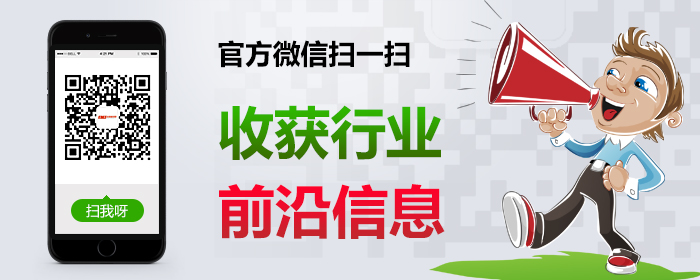
